Package: robregcc 1.1
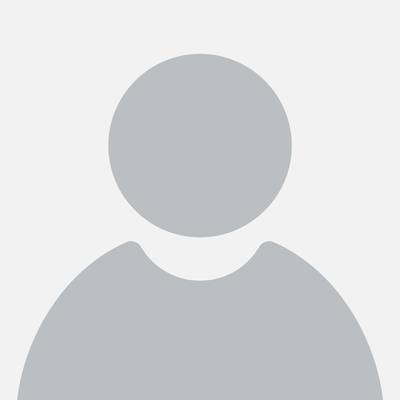
Aditya Mishra
robregcc: Robust Regression with Compositional Covariates
We implement the algorithm estimating the parameters of the robust regression model with compositional covariates. The model simultaneously treats outliers and provides reliable parameter estimates. Publication reference: Mishra, A., Mueller, C.,(2019) <arxiv:1909.04990>.
Authors:
robregcc_1.1.tar.gz
robregcc_1.1.zip(r-4.5)robregcc_1.1.zip(r-4.4)robregcc_1.1.zip(r-4.3)
robregcc_1.1.tgz(r-4.4-x86_64)robregcc_1.1.tgz(r-4.4-arm64)robregcc_1.1.tgz(r-4.3-x86_64)robregcc_1.1.tgz(r-4.3-arm64)
robregcc_1.1.tar.gz(r-4.5-noble)robregcc_1.1.tar.gz(r-4.4-noble)
robregcc_1.1.tgz(r-4.4-emscripten)robregcc_1.1.tgz(r-4.3-emscripten)
robregcc.pdf |robregcc.html✨
robregcc/json (API)
# Install 'robregcc' in R: |
install.packages('robregcc', repos = c('https://amishra-stats.r-universe.dev', 'https://cloud.r-project.org')) |
Bug tracker:https://github.com/amishra-stats/robregcc/issues
- simulate_robregcc - Simulated date for testing functions in the robregcc package (sparse setting).
- simulate_robregcc_nsp - Simulated date for testing functions in the robregcc package (non-sparse setting).
- simulate_robregcc_sp - Simulated date for testing functions in the robregcc package (sparse setting).
Last updated 4 years agofrom:0ea849d8c9. Checks:OK: 1 ERROR: 8. Indexed: yes.
Target | Result | Date |
---|---|---|
Doc / Vignettes | OK | Nov 06 2024 |
R-4.5-win-x86_64 | ERROR | Nov 06 2024 |
R-4.5-linux-x86_64 | ERROR | Nov 06 2024 |
R-4.4-win-x86_64 | ERROR | Nov 06 2024 |
R-4.4-mac-x86_64 | ERROR | Nov 06 2024 |
R-4.4-mac-aarch64 | ERROR | Nov 06 2024 |
R-4.3-win-x86_64 | ERROR | Nov 06 2024 |
R-4.3-mac-x86_64 | ERROR | Nov 06 2024 |
R-4.3-mac-aarch64 | ERROR | Nov 06 2024 |
Exports:classoclasso_pathcoef_cccpsc_spplot_cvplot_pathplot_residrobregcc_optionrobregcc_simrobregcc_sim2robregcc_sp
Dependencies:magrittrMASSRcppRcppArmadillo